Sequencing the human genome, transitioning to electronic health records and digitizing pathology images have all contributed to advancements in data generation and analysis that have catapulted science and medicine forward. But the true synthesis of this information—and more, including social media posts, drug databases, clinical trials and even text from clinical notes—is now possible, thanks to powerful algorithms and the collaboration of computational biomedicine experts and curious clinicians. This is emblematic of the promise of convergent science.
“We created the Department of Computational Biomedicine to address disparities in health through studies that use real-world data and to bring experts that can advance the methods needed to deal with such high-volume, content-rich data.”
– Graciela Gonzalez-Hernandez, PhD
AI in Action
AI and machine learning can already enable identification of pancreatic cancer before it’s detected on CT scans, help with early differential diagnosis of often underrecognized cardiac diseases and triage stroke patients to reduce length of stay.
Machine learning offers a huge opportunity to enhance the precision of clinical research and advance the data science infrastructure of Cedars-Sinai Cancer by supporting multimodal data processes through applications such as Molecular Twin. AI can also be used to provide the data that helps promote cancer clinical trials enrollment and engagement of minorities and underrepresented populations, such as the local Korean and LGBTQ+ communities.
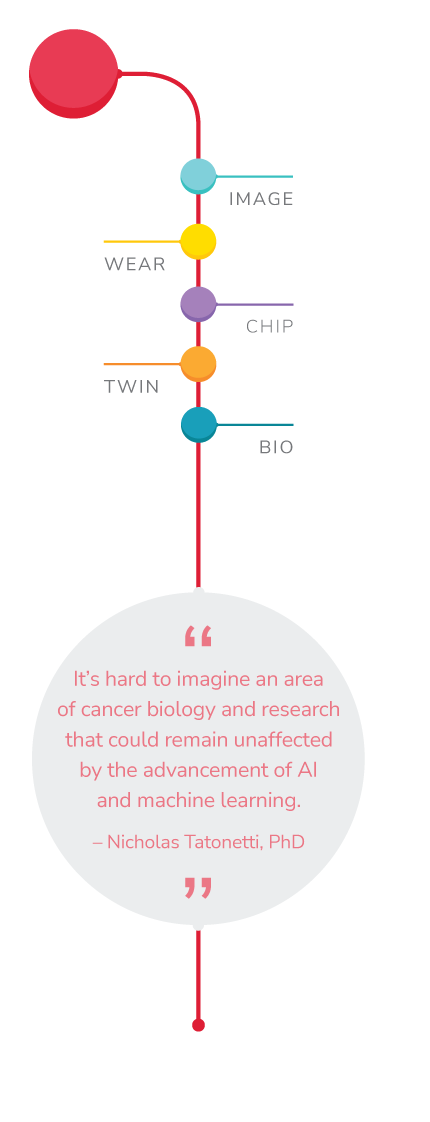